Stochastic interpolants have emerged as a powerful mathematical tool that bridges the gap between flows and diffusions in various scientific and engineering disciplines. By providing a unified framework, they enable researchers and practitioners to analyze complex systems with greater accuracy and efficiency. This article delves into the intricacies of stochastic interpolants, exploring their applications, mathematical foundations, and relevance in modern science.
As we navigate through this comprehensive guide, you will uncover the essential components of stochastic interpolants, their role in bridging flows and diffusions, and their significance in diverse fields. Whether you are a researcher, engineer, or simply curious about the mathematics behind complex systems, this article is designed to provide you with a deep understanding of the subject.
By the end of this exploration, you will gain insights into the practical applications of stochastic interpolants, their potential to revolutionize scientific research, and the future directions of this fascinating field. Let's embark on this journey together.
Read also:Fryd Lab Test
Table of Contents
- Introduction
- What Are Stochastic Interpolants?
- Mathematical Foundations
- Unifying Flows and Diffusions
- Applications in Science
- Real-World Examples
- Challenges and Limitations
- Future Directions
- Conclusion
- References
What Are Stochastic Interpolants?
Stochastic interpolants represent a mathematical concept that facilitates the interpolation of random variables across time and space. They are particularly useful in modeling systems where uncertainty plays a significant role. By combining elements of probability theory and interpolation techniques, stochastic interpolants provide a robust framework for analyzing complex phenomena.
Definition and Importance
The core idea behind stochastic interpolants lies in their ability to estimate intermediate values within a stochastic process. This is crucial for understanding the dynamics of systems that evolve over time, such as weather patterns, financial markets, and biological processes. The importance of stochastic interpolants stems from their versatility and applicability across multiple domains.
Key Features
- Ability to handle uncertainty and randomness.
- Integration of interpolation techniques with probability theory.
- Scalability to high-dimensional problems.
Mathematical Foundations
The mathematical underpinnings of stochastic interpolants are rooted in advanced concepts from probability theory, stochastic calculus, and functional analysis. Understanding these foundations is essential for harnessing the full potential of stochastic interpolants in practical applications.
Stochastic Processes
At the heart of stochastic interpolants lies the concept of stochastic processes, which describe the evolution of random variables over time. These processes can be continuous or discrete, depending on the nature of the system being modeled. Common examples include Brownian motion and Markov chains.
Interpolation Techniques
Interpolation methods, such as polynomial interpolation and spline interpolation, are combined with stochastic elements to create stochastic interpolants. These techniques ensure that the interpolated values remain consistent with the underlying probability distribution of the system.
Read also:Secretstars Lilu
Unifying Flows and Diffusions
One of the most significant contributions of stochastic interpolants is their ability to unify flows and diffusions into a single framework. This unification allows for a more comprehensive understanding of systems that exhibit both deterministic and stochastic behaviors.
Flows
Flows refer to the deterministic movement of particles or quantities within a system. They are governed by differential equations and are often used to model physical phenomena such as fluid dynamics and celestial mechanics.
Diffusions
Diffusions, on the other hand, describe the random movement of particles or quantities. They are typically modeled using stochastic differential equations and are prevalent in fields such as finance and biology.
Applications in Science
The versatility of stochastic interpolants makes them applicable across a wide range of scientific disciplines. From physics to finance, their ability to model complex systems has proven invaluable.
Physics
In physics, stochastic interpolants are used to study turbulent flows, quantum mechanics, and statistical mechanics. Their capacity to handle uncertainty makes them ideal for analyzing systems with inherent randomness.
Finance
In the financial sector, stochastic interpolants are employed to model asset prices, interest rates, and risk management strategies. They enable financial analysts to make more accurate predictions and informed decisions.
Real-World Examples
Several real-world examples demonstrate the practical utility of stochastic interpolants in solving complex problems.
Weather Forecasting
Stochastic interpolants are used in weather forecasting to predict temperature, precipitation, and wind patterns. By incorporating uncertainty into their models, meteorologists can provide more reliable forecasts.
Biological Systems
In biology, stochastic interpolants help model the spread of diseases, population dynamics, and genetic mutations. Their ability to account for randomness ensures more accurate representations of biological processes.
Challenges and Limitations
Despite their many advantages, stochastic interpolants are not without challenges and limitations. Understanding these constraints is essential for their effective application.
Computational Complexity
One of the primary challenges associated with stochastic interpolants is their computational complexity. High-dimensional problems can be computationally expensive and time-consuming to solve.
Data Requirements
Stochastic interpolants often require large amounts of data to produce accurate results. In cases where data is scarce or noisy, their performance may be compromised.
Future Directions
The future of stochastic interpolants holds great promise, with ongoing research aimed at overcoming existing challenges and expanding their applications.
Advancements in Computational Techniques
Advances in computational techniques, such as machine learning and artificial intelligence, are expected to enhance the efficiency and accuracy of stochastic interpolants. These technologies can help mitigate computational complexity and improve scalability.
Integration with Other Fields
Further integration of stochastic interpolants with other fields, such as artificial intelligence and quantum computing, could lead to groundbreaking discoveries and applications.
Conclusion
Stochastic interpolants represent a powerful mathematical framework for unifying flows and diffusions in complex systems. Their ability to handle uncertainty and randomness makes them indispensable tools in various scientific and engineering disciplines. By addressing challenges and embracing future advancements, stochastic interpolants have the potential to revolutionize the way we understand and model the world around us.
We invite you to engage with this article by sharing your thoughts, asking questions, or exploring related topics. Together, we can continue to deepen our understanding of stochastic interpolants and their applications.
References
1. Kloeden, P. E., & Platen, E. (1992). Numerical Solution of Stochastic Differential Equations. Springer.
2. Oksendal, B. (2003). Stochastic Differential Equations: An Introduction with Applications. Springer.
3. Gardiner, C. W. (2009). Stochastic Methods: A Handbook for the Natural and Social Sciences. Springer.
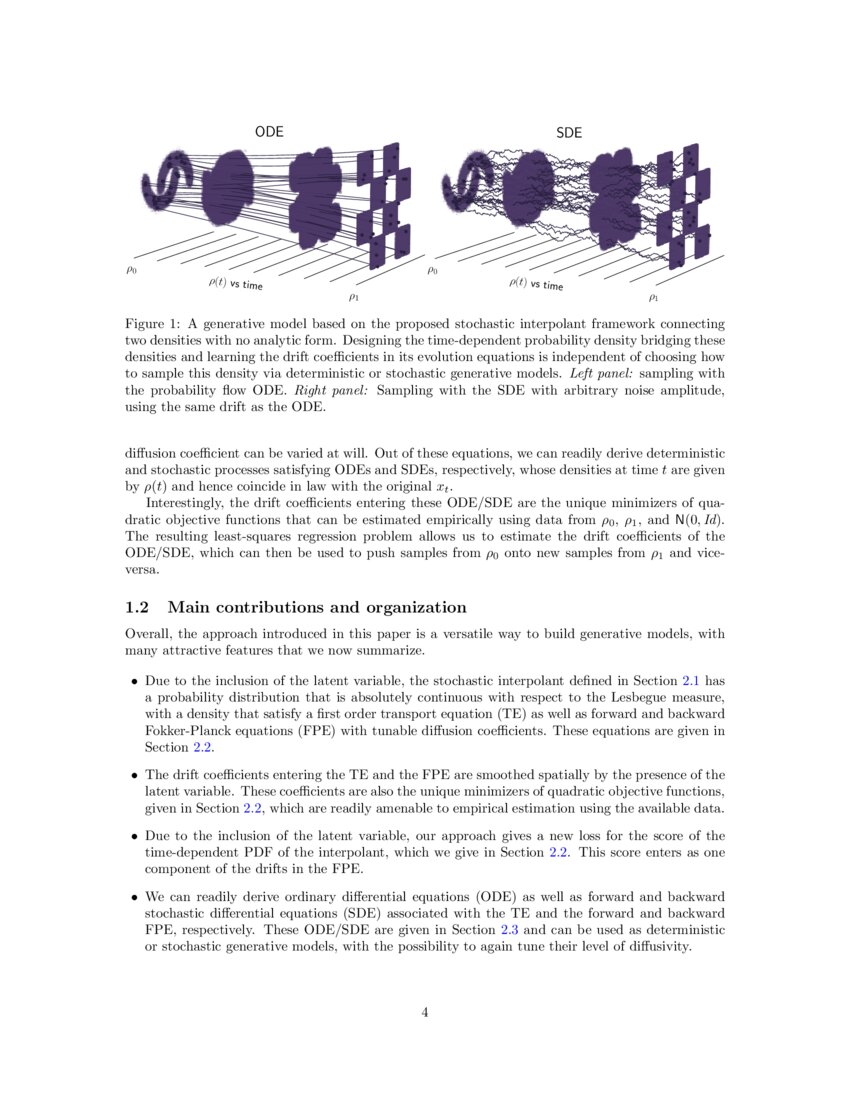
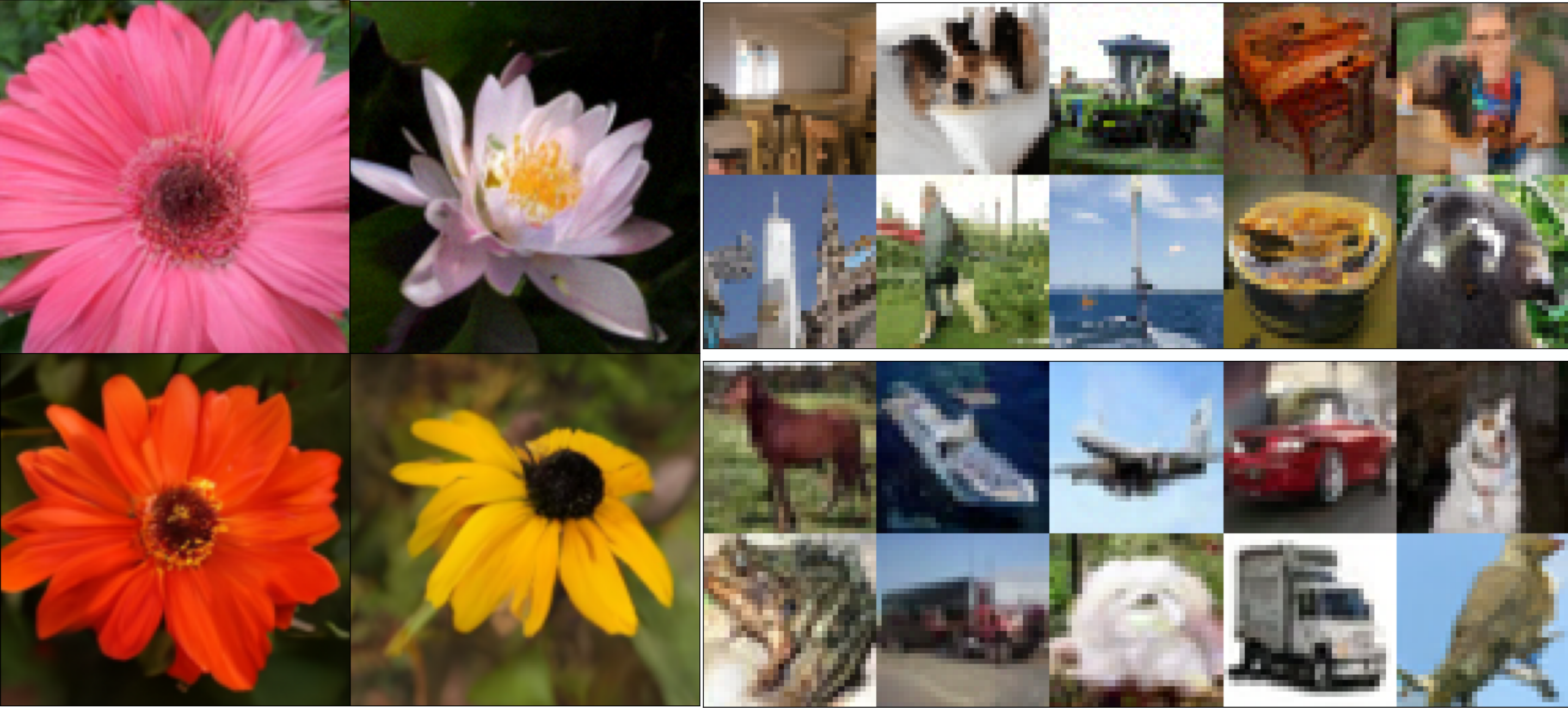